Can computers recognise (musical) beauty? Can we? Does beauty even exist? And if it does, can we project such notion onto computational systems so that they can categorise artifacts accordingly, or so that they can create artifacts that abide by it? How?
In the vernacular sense ascribed to it, aesthetics is the study of what is beautiful. However, in philosophy of the art, aesthetic has evolved to encompass the not-so-beautiful, the unpleasant and the ugly, even. Thus, aesthetics is no longer associated to an objective measure of beauty and pleasantness.
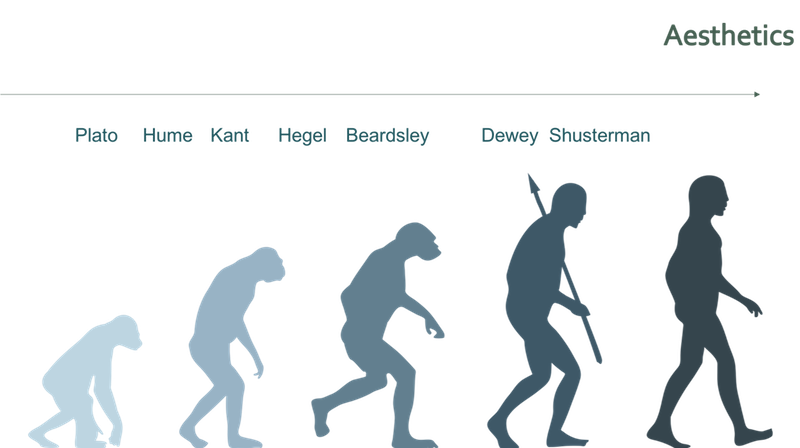
In the context of creative AI, a system is not devoid of the assumptions of its designer, implicitly embedding her aesthetic/artistic objectives and preferences. Nevertheless, it is important to consider how one can model aesthetic evaluation in such systems. Computational aesthetics is, in fact, the theory, practice and applications of aesthetics in computing. There can be two ways to approach it:
One considers the aesthetic value that a computational process (e.g., an algorithm) or computational product (e.g., an algorithmically generated artwork) holds for a human.
The other is concerned with the ability of computational systems to judge the aesthetic value of their own or someone else’s creative product.
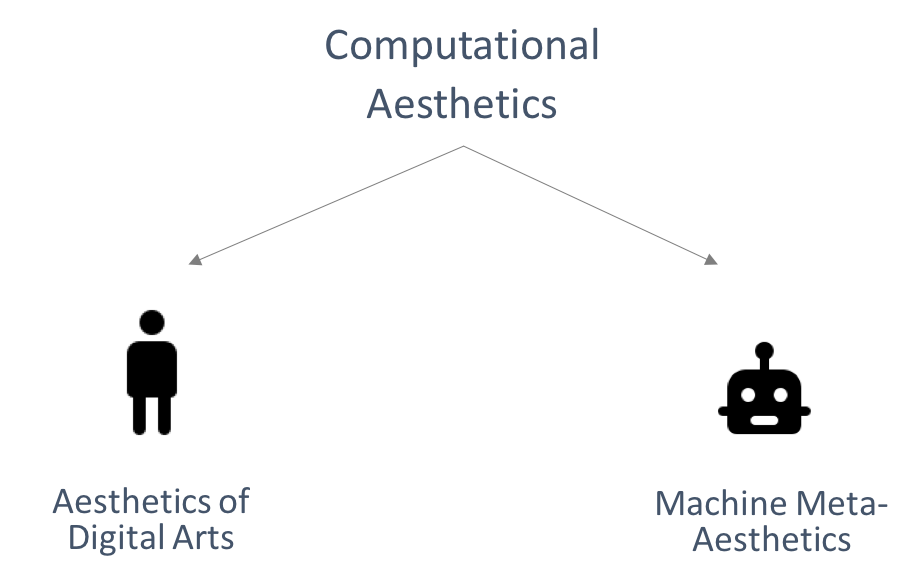
Creative AI is concerned with the latter, to the extend that it is interested in building computationally creative systems.
Many methods have been proposed to implement computational measures of aesthetic value. These can be generally categorised according to whether they are based on:
- Information/Complexity Theory
- Fractal Dimension and Geometry
- Psychology and Gestalt
- Grammar
- Biology/Evolution Theory
- Connectionism/Neural Networks
For a comprehensive review of these, the reader is encouraged to consult this article.
Although computational aesthetics has been the object of attention primarily in creative AI application to poetry, arts, graphics and design, generative music systems have not been immune to this discourse.
For example, the Audio Oracle (AO) is an algorithm that applied information dynamics in the context of human-machine improvisation and sound design. It is based on the assumption that a pleasing aesthetic experience is conditioned upon a balance between order and complexity. Such assumption is a heritage of Birkhoff’s aesthetic measure M:
where C and O are Shannon’s entropy and conditional entropy
, respectively. The AO defines Information Rate (IR) similarly to the conditional entropy (above), albeit using a compression algorithm C which measures the number of bits required to represent the data with or without knowledge of the past:
The AO is a graph structure on indexed segments of a recording’s audio features, which could be an alternative to spectral clustering and recurrence analysis. However, it is not used for determining structural segments, but rather to recombine sections and sub-clips of the audio analysed, with human-machine interaction as a goal.
Some post-modern musical expressions consider repetition and redundancy as axioms of their aesthetic stance. It is often the case that the relation between novelty and expectation as expressed by the IR is deliberately violated, for artistic purposes. A few examples might be minimal music, post-minimalism, process music, drone music, sound art, aleatoric music, free improvisation, noise, glitch, microsound, lowercase and so forth.
In the case of CCCC’s Test Tube Fantasy Part One, for example, the visualisation of the optimal oracle might fail to give useful insights, although it yields an interesting visual result, perhaps an unintended artistic by-product of the total sonic redundancy of the piece:
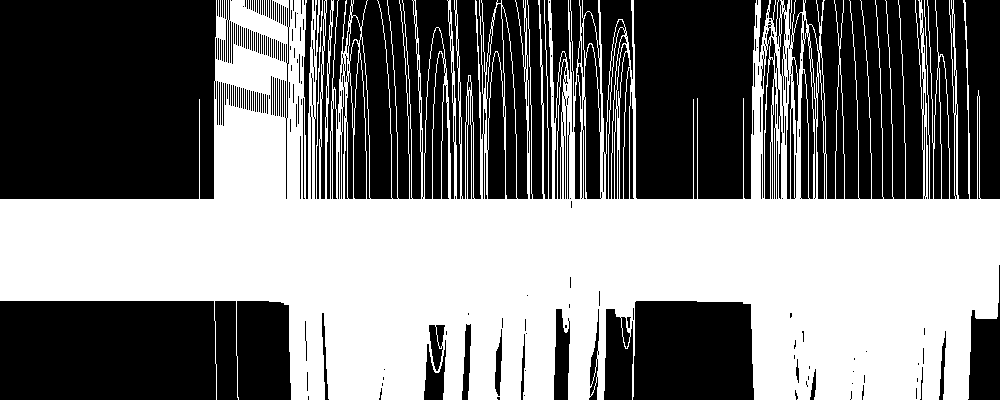
AO is not the only method that has been used to evaluate the aesthetic value of musical works. Amongst the geometrical approaches, fractal dimension has attracted considerable attention.
Zipf’s Law has been used to calculate the aesthetic measure of genres and to infer conclusions from such analysis. It expresses the occurrence frequency of an ranked event e, using the ratio
with a being close to 1. This law states that the probability of an event occurring is inversely proportional to its frequency of occurrence. According to certain claims, beautiful music should exhibit a similar behaviour, with respect to musical parameters such as pitch, duration, intervals and so forth.
Not unlike the previous observation regarding IR, a statistical distribution of considered events has little to say regarding the temporal dependencies of the events themselves, and how these affect the perception of the work. Furthermore, frequency distribution is not informative when the artistic goal/value of the work is embedded in the use of a minimal (sometimes exclusive) palette of sounds or in the absence of meter (e.g., drone music). The images below show the slope with respect to in-between onset detection time durations for Steve Reich’s Pendulum Music and for Aphex Twin’s Spots from Selected Ambient Works Volume II. A frequency distribution abiding by Zipf’s Law would have had to yield a slope of approximately -1, which is clearly not the case for the works considered below.
Pendulum Music | Spots |
---|---|
![]() |
![]() |
The more one examines post-modern musical expressions, the more unreasonable it becomes to conclude that a work’s aesthetic value can be reduced to a quantitative analysis, isolated from its socio-political context.
It is evident that there are no boiler plate and generalisable notions of beauty, nor corresponding computational methods that can abstract it, to date. In the awareness of the existing gap between aesthetic notions in philosophy of the art, psychology and computer science, it is, nevertheless, crucial to continue to investigate the field of computational aesthetics.
Thanks to the introduction of a new factor (the machine) in the broader discourse on aesthetics, new opportunities to question our cherished notions of beauty, pleasantness, order and purpose become available.
If we are to hope for truly creative AI systems, it is thus paramount that the field of computational creativity embraces the complexity of the subject matter, and of the arduous task of integrating a theory of experience (aesthetics) and a method of abstraction (computation).
About the Author:
![]() |
Stefano Kalonaris is a creative technologist, musician and researcher who specialises in Interactive Music Systems, and Performance. He holds a PhD from the Sonic Arts Research Centre, Queen’s University Belfast and he is currently a postdoctoral researcher in Music Informatics at the RIKEN Center for Advanced Intelligence Project (AIP), Japan. He has worked on networked music performance, and improvisational interfaces for human-computer interaction, and generative systems for music creation. Stefano has performed at international venues and festivals, including Sonorities, Diffrazioni, Café Oto, STEIM, the London Jazz Festival and many others. |
---|---|